
- This event has passed.
Equity in IDEA: Comparing the Current IDEA Equity Requirements and Understanding the Proposed Changes for Significant Disproportionality
August 10, 2016 @ 5:00 pm - 6:00 pm EDT
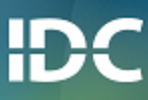
Register to join the IDEA Data Center (IDC) on August 10, 2016 from 1:00-2:00 PM EST for a webinar on Equity in IDEA: Comparing the Current IDEA Equity Requirements and Understanding the Proposed Changes for Significant Disproportionality. Learn about the various equity requirements in IDEA, including Disproportionate Representation (Indicators B9 and B10), Significant Disproportionality, and Significant Discrepancy in Discipline (Indicator B4). Find out about the U.S. Department of Education’s Notice of Proposed Rule Making (NPRM) Promoting Equity in IDEA and what states need to do differently for significant disproportionality if the NPRM moves forward. Finally, gain a better understanding of the similarities and differences among these various equity requirements.
The webinar will be recorded for those unable to attend.